Mass credit defaults to ensue after moratorium ends? Banks not in a pleasant position
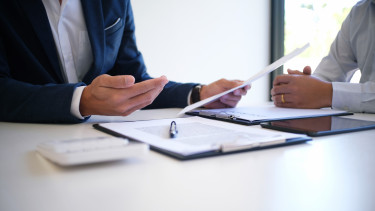
We are getting closer to the end of the loan repayment moratorium period, there are a lots of forecasts regarding the growth of insolvency among corporate and retail customers. What are the expectations regarding insolvency numbers in Europe and Hungary? Are there any differences worth mentioning?
Gábor Győrfi: Yes, we are getting closer to the expected end of moratorium periods. However, during an economic recovery period it is also possible
that governments will prolong some measures in order to give specific sectors and groups of debtors more time to get back on track.
So governments may consider not just simply ending the moratoriums, but allowing a step-by-step approach with differentiated rules for more vulnerable groups of debtors.
Insolvency numbers are not easy to estimate, especially when during a moratorium period the banks have limited ways to learn about the changing payment capabilities, willingness to pay, and spending habits of their customers therefore they need to be prepared even for significant changes, even for worst-case scenarios.
The European Central Bank (ECB) has already tried to explore in a special report called 'NPL Outlook Europe 2021' how prepared banks are for a significant raise in NPL. Unfortunately, the forecasting capabilities are already limited, so several big players on EU banking market don’t know what to expect, which makes it difficult for them to prepare for such a cloudy future.
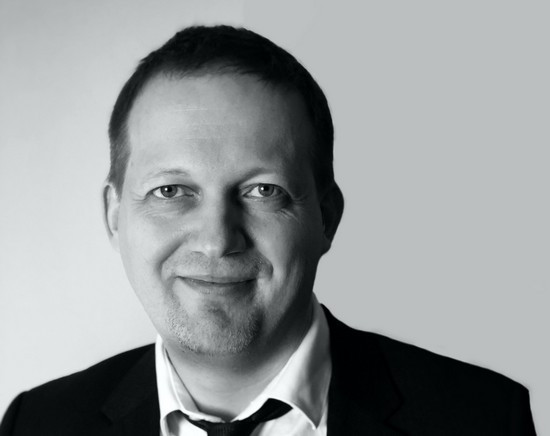
As regards corporate customers, which sectors are the most vulnerable ones? Which ones are running the biggest risk of insolvency and why?Which countries’ bank system is affected the most by these vulnerable sectors?
G.Gy.: Lockdowns have an obvious impact on prominent sectors like tourism, heavily affect hotels, restaurants and all their suppliers. Also, there can be many other sectors where risks stem mainly from the sheer size of the companies rather than their area of operation. Even the smallest of changes in customer preferences can easily ruin family businesses, micro and small companies regardless of the sector they are in.
Governments need to carefully consider measures country by country and sector by sector, and act quickly to bail out such vulnerable segments.
On the retail side, how much do we have to worry about an overwhelming number of credit defaults? How effectively do you think savings accumulated by households during the pandemic can mitigate the risk of default?
G.Gy.: The risks are clearly great also on the retail side, where
defaults could exceed the size of savings.
Once the moratoriums are over, the most vulnerable groups in banks’ portfolios will be those that had turned to banks for loans due to a shortage of savings and liquid resources. Those with stable income and savings stand a greater chance of repaying their debts even after the end of the moratorium period.
Changes to customers’ spending and paying habits and preferences could still alter this logic, though. I’m referring to changes induced by the lockdown measures, e.g. focus shifted to online purchases and families retiming or rescheduling investments (consumer durables, summer vacation, etc.).
To what extent can machine learning or other technologies help effectively manage the NPL portfolios and reduce the number of insolvent customers? Are these technologies capable of anticipating such defaults?
G.Gy.: It’s no longer a question that there is a huge potential in analytics and technology-supported NPL management. On top of the legacy NPL portfolios now we expect a significant number of new defaults in the portfolios. The banks would need to understand their debtors and adapt to the new profiles, behaviour patterns within a short period of time in order to be able to handle them effectively. These changing and risky times will not allow for slow reactions, hesitation, experimenting, they rather require quick and steadfast decisions with commitment. Here a state-of-the-art support technology and data analytic capability are indispensable. An ML-based modelling and forecasting of the customers’ financial situation and behavioural patterns can be a great asset to implement more cost-efficient strategies. Contrary to beliefs, this does not imply distancing from customers. Quite the contrary, debtors will find that they and their cases are managed in a much more tailor-made fashion. The benefits of a technology-driven liability management have already been known, but they will be more prominent and more tangible than ever during these difficult times.

Cover photo: Getty Images